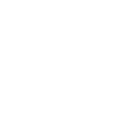
AI lab TL;DR | Martin Senftleben - How Copyright Challenges AI Innovation and Creativity
Manage episode 455797900 series 3480798
🔍 In this TL;DR episode, Martin Senftleben (Institute for Information Law (IViR) & University of Amsterdam) discusses how EU regulations, including the AI Act and copyright frameworks, impose heavy burdens on AI training and development. The discussion highlights concerns about bias, quality, and fairness due to opt-outs and complex rights management systems, questioning whether these rules truly benefit individual creators. A proposal is made to focus regulatory efforts on the market exploitation phase of AI systems, ensuring compensation flows back to creative industries and authors through well-managed redistribution mechanisms.
📌 TL;DR Highlights
⏲️[00:00] Intro
⏲️[01:04] Q1-How does the EU's current approach to AI training and copyright hinder innovation and fair pay for authors?
⏲️[04:53] Q2-What’s your alternative to balance author compensation and AI development?
⏲️[06:50] Q3-Why is output-based remuneration better for creators, AI developers, and society?
⏲️[09:23] Wrap-up & Outro
💭 Q1 - How does the EU's current approach to AI training and copyright hinder innovation and fair pay for authors?
🗣️ "What policymakers try to do in this space where we try to reconcile AI innovation with traditional copyright goals is: first of all, of course we want the best AI systems and we want the least biassed AI systems."
🗣️ "The regulation puts a heavy, heavy burden on AI training by requiring to take into account rights reservations, the so-called opts-outs."
🗣️ "You might not get the best AI systems if you put all these burdens on the AI training process."
🗣️ "The moment you allow rights holders to opt out and to remove certain resources from training, then of course you no longer know whether you get the least biassed AI systems."
🗣️ "The simple fact that a big creative industry right holder receives some extra money doesn't mean that this money is passed on to the individual authors really doing the creative work."
💭 Q2 - What’s your alternative to balance author compensation and AI development?
🗣️ "If we imagine a regulation that leaves this development phase totally unencumbered by copyright burdens, you give lots of freedom for AI developers to use all the resources they think are necessary."
🗣️ "Once we have these fully developed, high potential AI systems and these systems are brought to the market, (...) You place a tax, a burden on the AI systems, not at the development stage, but at the moment where they are exploited in the marketplace.”
🗣️ "The money finally flows back in the form of compensation to the creative industry and individual authors."
💭 Q3 - Why is output-based remuneration better for creators, AI developers, and society?
🗣️ "From a European perspective, it's quite easy to propose that this should be collecting societies because we have a very well developed system of collective rights management in the area of copyright."
🗣️ "In the case of AI output, you can also use data from the systems itself: to which extent is a certain style, a certain genre prominent in prompts that users enter? What type of AI output is generated and to which extent does it resemble certain pre-existing human works and creations? What is the market share on the more general market for literary, artistic expression and so on?"
🗣️ "Traditionally, repartitioning schemes have a split between money that is directly given to individual authors and money that is given to the industry.We have a guarantee that a certain percentage of the money will directly reach the individual authors and performers, and will not stay at industry level exclusively."
📌 About Our Guest
🎙️ Martin Senftleben | Institute for Information Law (IViR) and University of Amsterdam
🌐 Article | Win-win: How to Remove Copyright Obstacles to AI Training While Ensuring Author Remuneration (and Why the European AI Act Fails to Do the Magic)
🌐 Martin Senftleben
linkedin.com/in/martin-senftleben-2430aa5b
Martin Senftleben is Professor of Intellectual Property Law and Director, Institute for Information Law (IViR), University of Amsterdam. His activities focus on the reconciliation of private intellectual property rights with competing public interests of a social, cultural or economic nature. He publishes extensively on these topics and lectures across the globe.
#AI #ArtificialIntelligence #GenerativeAI
32 episod